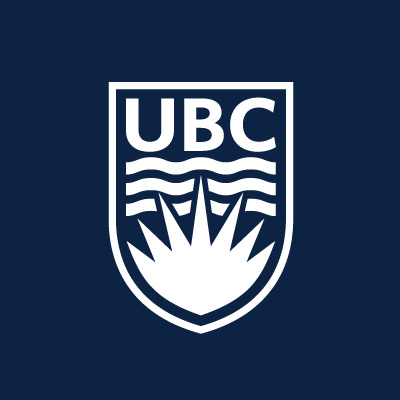
University of British Columbia
2019–2025
2019–2025
2022–2025
2020–2020
2017–2017
2014–2014
2009–2011
2005–2009
Application of neural networks and deep learning architectures for structural analysis and monitoring.
Implementing computer vision techniques for visual inspection and structural damage detection.
Developing intelligent systems for real-time monitoring of civil infrastructure.
High-resolution image segmentation is essential in structural health monitoring (SHM), enabling accurate detection and quantification of structural components and damages.
Additively manufactured components often contain volumetric defects that significantly impact mechanical and fatigue properties across various material systems.
In this study, two binary versions of the Water Strider Algorithm (WSA) are proposed and applied to optimal feature selection in classification problems.
In this article, an open-source ABAQUS/MATLAB-based framework is developed for the bending-induced buckling design of variable-stiffness (VS) composite cylinders.
This study introduces a novel convolutional neural network (CNN)‐based approach for structural health monitoring (SHM) that exploits a form of measured compressed response data.
Inspired by swarm intelligence in nature, a new control method, known as swarm-based parallel control (SPC), is proposed in this study.